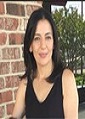
Hakima Ibaroudene
Southwest Research Institute, USA
Biography
Hakima Ibaroudene holds a B.S. and M.S. in Electrical Engineering from the University of Texas in San Antonio. She is a Group Leader in Research Development, a non-profit applied research organization. She has published more than five papers in reputed journals and served as session chair member for the International Telemetry Conference in 2017 and 2018.
Abstract
We demonstrate an approach to predicting cancer cellularity which uses a combination of weakly- and strongly-labeled data to train a convolutional neural network, where the cellularity scores serve as weak labels and segmentation labels serve as strong labels. By providing segmentation labels for only a small subset of the dataset, we add constraints to the locations of activations at the end of a fully convolutional neural network which predicts cellularity using a global average pool. We utilize a recent neural network architecture, Squeeze-Excitation networks, which reweight feature vectors according to global content in order to refine local predictions. Our method won the BreastPathQ challenge, with our best submission earning an average Pk of .941. Our method is also extremely fast, processing each patch in approximately 19ms, and a whole slide in a matter of minutes